DeepSeek Explored
Over the weekend of the 25th of January, 2025, the word DeepSeek emerged into the consciousness of the Internet and took it by storm. For many consumers, the company, the language model, the app appeared to have jumped out of nowhere and instantly became a huge hit.
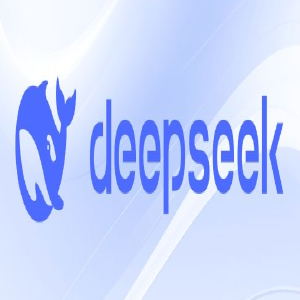
For those well versed with the players in the artificial intelligence community, the name was somewhat familiar, though it was not commonly seen in the media, which has long been overshadowed by big names such as OpenAI (ChatGPT model family), Meta (Llama model family) and Google (Gemini model family).
What was different about DeepSeek and one of the main causes of it becoming a sensation is that it delivered amazing reasoning results. In the synthetic tests, it delivered test results as good as, and in some cases surpassing, the latest models from OpenAI and Google.
What made it stand out was that it delivered those results while being being really, really efficient. Some people were claiming it was up to 90 or 95% more efficient, however that is a number to take with a grain of salt as broad averages do show greater efficiency, but not always to that extent.
What did DeepSeek do differently?
It was able to distill a lot of that information into an architecture (called MoE), which stands for Mixture of Experts. This means that the model is able to target its reasoning to specific parts of the prompt, rather than trying to solve the entire prompt at once. The immediate technical consequence is that the model does not need to load all of its parameters into memory, which means that running inference on it requires less transformer operations. All this adds up to a model that is much more efficient.
While the architecture is brilliant, it’s also important to keep in mind that it is built on the shoulders of the open source models that were trained from source material before it. It’s ability to take a running leap meant that it was able to use a pretrained model and optimise it. In a humourous turn of events, the DeekSeek R1 model sometimes refers to itself as “OpenAI” or “GPT-4” in its responses.
Additionally, the team at DeepSeek were constrained by compute resources, meaning they were forced to find every possible way to optimise the model. With geopolitical tensions, the US has been very strict with export controls on the powerful Nvidia H100 compute chips, which are used to train and run inference on large language models. The team found a way to use the slower Nvidia H800 chips to train the model by writing custom code and bypassing the CUDA architecture. This gave them additional flexibility to optimise their model design. This is one of the key factors for being able to train the model on 2,048x H800 servers for an estimated cost of US$5M-$6M.
The team themselves have been clear that this is only for the final training phase and excludes all previous experiments and ablations. To their credit, they have been transparent about the process and the results, including publishing the paper and the model itself to HuggingFace. Other AI labs across the world are trying to replicate the results to verify the claims.
Number 1 on the app stores
As a result of being so fast and cheap, the DeepSeek app quickly rose to the top of the app stores, surpassing the stalwarts of ChatGPT and Google’s Gemini.
As consumers started playing with the application, they were able to interact with the model and see the results. This was a big deal for a lot of people, as it was the first time they were able to interact with a large language model that was not OpenAI, Meta, or Google. Not only that, the model was quite good and gifted to the world free by a Chinese company.
Western world’s reaction
For those in the financial markets, it was such a big deal that on Monday’s opening bell, Nvidia stock alone dropped by over $600 billion.
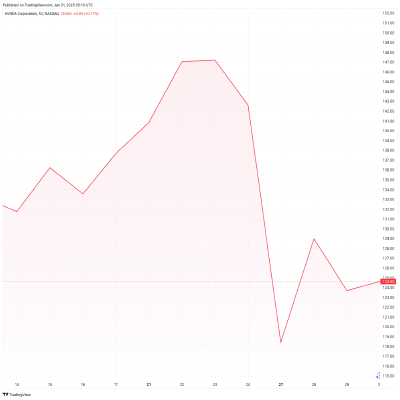
The question was asked: does the world need Nvidia at the same level of investment anymore?
As the markets battled to find the answer over the several days that followed, it was clear that this moment was a signficant fork in the road for AI technology.
The (short-term) future of AI
No one can predict the long term future of AI, however the short term future has some interesting implications.
What DeepSeek has done is to show that it is possible to create a large language model that is both fast and cheap. For many workloads, this is more than enough to replace the need for a traditional, cloud-based, large language model.
Putting a bet on the future of AI, I would expect there to be more need for relatively lower-powered inference on edge devices, rather than centralising all inference on large cloud compute resources.
This is something that Nvidia has recognised and is investing heavily in, with the Nvidia Edge computing platform.
If we can run small parameter models on edge devices, then we can do a lot of inference very quickly, eliminating the need for network roundtrip latency.
In addition to that, the democratisation of AI means that more people are able to access it and more people are able to build on it. This inevitably leads to more innovation and more competition, which is good for the industry.